Project IntelligEnt
The IMMS researched assistance systems for chip designers: Machine Learning improves design and test methods for integrated analogue/mixed-signal systems.
Designers‘ know-how for mixed analogue/digital systems difficult so far to automate
Crucial in the creation of microelectronic and micro-electro-mechanical systems (MEMS) is the design engineer's knowledge and experience. The development of such systems has long been researched, with improvements and ever more sophisticated automatic design methods being achieved. It has to be said that the engineer's knowledge from experience is not easy to represent visually so that it can be used for automatic design as would be the case if the systems were purely digital. In consequence, systems that are analogue and complex or are mixed analogue/digital are often less than perfect or have incongruencies like poor layout or incorrect test limits which come to light only later, often not until they interact with the components they are serving. More time and money thus has to to be invested at both the design stage and that of validation of the systems after fabrication.
Machine learning at the system design stage for major reductions in cost and risk
Therefore, the IntelligEnt group formed by IMMS and Ilmenau TU have developed application-oriented machine learning concepts in the design of microelectronic devices which can be tacked on to existing methodology and tools. The aim was to make use of the huge potential of machine learning for continued practical and research progress, dramatically reducing both the costs and the risks associated with system design.
This is possible if the incongruencies are found early and obviated to maximum effect. There are many areas in which machine learning has the edge over humans, among them being pattern recognition. If an automated design and characterisation process is integrated into the system design procedure it may, by recognising structures, reduce the total sum of data, sniff out anomalies and greatly improve the existing solution. In the IntelligEnt project, the machine learning algorithms are seen as tools with uses such as regression and classification based on deep learning methodology or for detection of outliers based on self- and semi-supervised learning.
The IntelligEnt work focussed on critical steps in the system design:
- Modelling – creating models of predicted behaviour: Critical for the design quality is the creation of models of the system components and/or the IP. The idea is that integrating features like power consumption and operational areas into system level models will in the end be automated because the system is capable of learning.
- Creating functions when designing analogue/mixed signal circuits: The structure or the topology is what determines how well a mixed analogue/digital circuit performs. To get computers to optimise the structure, a procedure for structure recognition and adaptation has been developed.
- Designing the chip manufacturer‘s construction plan at the analogue/mixed signal circuit layout stage: Layouts that are technically correct may still have incongruencies, among them substrate coupling, field transistors and mismatch. With Ilmenau TU, we have developed an AI-based anomaly detection method to automatically detect non-proven and potentially faulty locations in layouts. Our relatetd plugin for the free design tool KiCad is available on GitHub.
- Checking all steps and functions at pre-fabrication stage by simulation and verification: at each of the above stages, before the chip is fabricated, the system is checked, with the function groups increasing in size at each stage. The simulations carried out for this purpose make use of the models that have been extended by the machine learning methodology.
- Checking manufactured chips with absolute thoroughness by testing and characterisation: to date, optimisation of the test procedures and the choice of critical tests for MEMS and mixed signal systems have had to be done manually, which can mean that redundant tests are carried out. Machine learning algorithms are capable of revealing the factors which are interdependent, so that they can be taken account of. IMMS has developed a machine-learning-based method for analysing measurement data from
ASICs. It delivers the same results as a manual evaluation, but is 10 – 30 times faster.
Acronym / Name:
IntelligEnt / Artificial intelligence and machine learning for the design and verification of complex systemsDuration:2019 – 2020
Application:
|Integrated circuit technology (design| verification and test)Research field:Integrated sensor systems
Related content
Reference
Prof. Dr.-Ing. Patrick Mäder, Technische Universität Ilmenau
”For us, the cooperation shows how important IMMS is for a transfer of results from basic research into practice.”
Reference
Christian Paintz, Melexis
“Particularly in the evaluation of measurement data, IMMS has impressively demonstrated that a learning algorithm is on a par with manual evaluation – while saving a great deal of time. We are also continuing to pursue methods for circuit and layout analysis, as we see great research and application potential here as well.”
Machine-Learning im PCB-Entwurf: Open-Source zwischen Forschung, Potential und Alltag
Georg Gläser1.30. Konferenz des Fachverbandes für Design, Leiterplatten- und Elektronikfertigung (FED), 29. - 30. September 2022, Potsdam, Germany
1IMMS Institut für Mikroelektronik- und Mechatronik-Systeme gemeinnützige GmbH, Ehrenbergstraße 27, 98693 Ilmenau, Germany.Intelligente Layoutverarbeitung: KI für ASIC- und PCB-Layouts
Georg Gläser1. Julian Kuners1.Elektronik, 03.2022, 9. Februar 2022, Seite 42 - 45, ePaper: wfm-publish.blaetterkatalog.de/frontend/mvc/catalog/by-name/ELE
1IMMS Institut für Mikroelektronik- und Mechatronik-Systeme gemeinnützige GmbH, 98693 Ilmenau, Germany.Machine-Learning-basierte Messdatenanalyse für ASICs. Testen auf der Überholspur
Tom Reinhold1. Georg Gläser1.Elektronik, 01/02.2022, 26. Januar 2022, Seite 46 - 48, ePaper: wfm-publish.blaetterkatalog.de/frontend/mvc/catalog/by-name/ELE
1IMMS Institut für Mikroelektronik- und Mechatronik-Systeme gemeinnützige GmbH, 98693 Ilmenau, Germany.Trash or Treasure? Machine-learning based PCB layout anomaly detection with AnoPCB
Henning Franke1. Paul Kucera1. Julian Kuners1. Tom Reinhold2. Martin Grabmann2. Patrick Mäder1. Marco Seeland1. Georg Gläser2.2021 17th International Conference on Synthesis, Modeling, Analysis and Simulation Methods and Applications to Circuit Design (SMACD), Proceedings in: 423 Seiten, 140 x 124 mm, Slimlinebox, CD-Rom, ISBN 978-3-8007-5588-2, E-Book: ISBN 978-3-8007-5589-9, ieeexplore.ieee.org/document/9547913, 19 - 22 July 2021, Erfurt, Germany, online
1Technische Universität Ilmenau, Germany. 2IMMS Institut für Mikroelektronik- und Mechatronik-Systeme gemeinnützige GmbH, 98693 Ilmenau, Germany.
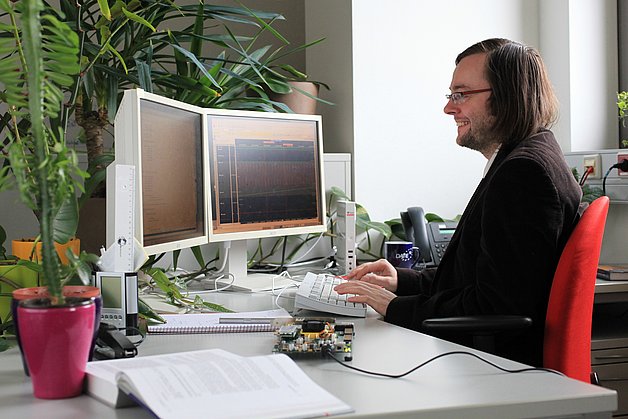
Event,
CiS Workshop 2023
Workshop Simulation & Design 2023 at CiS Forschungsinstitut für Mikrosensorik
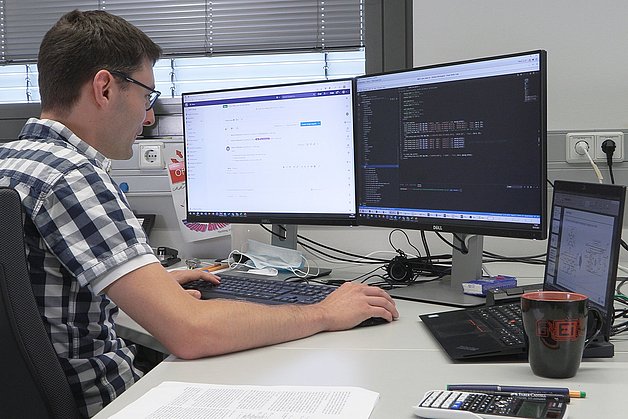
Event,
FED Conference
30th Conference of the German Association for Design, Printed Circuit Board and Electronics Manufacturing (FED)
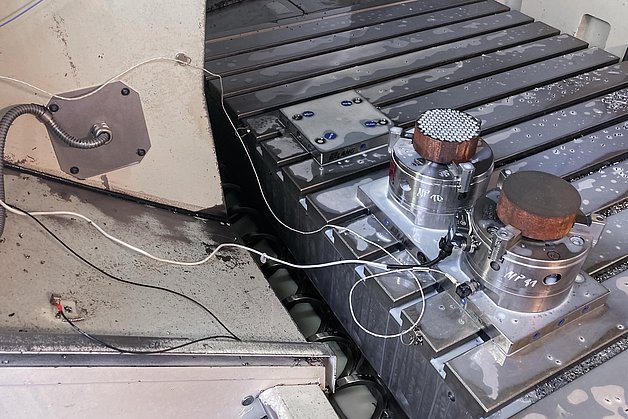
Event,
Forum Künstliche Intelligenz
Artificial Intelligence Forum of the German specialist media Elektronik, Elektronik automotive und Computer&AUTOMATION. The forum was cancelled by the organiser.
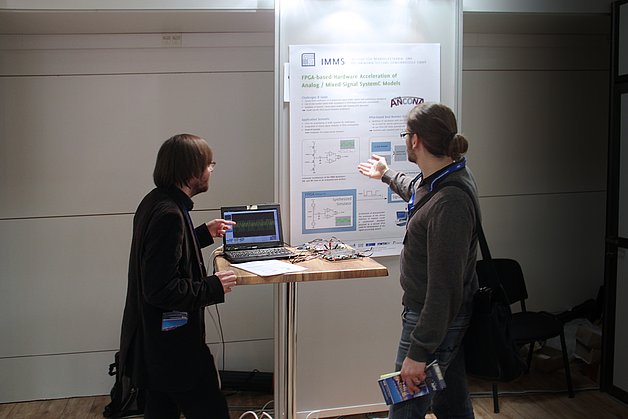
Event,
KI für KMU
KI für KMU – Prozesse optimieren mit künstlicher Intelligenz, Vortragsveranstaltung von Mittelstand 4.0
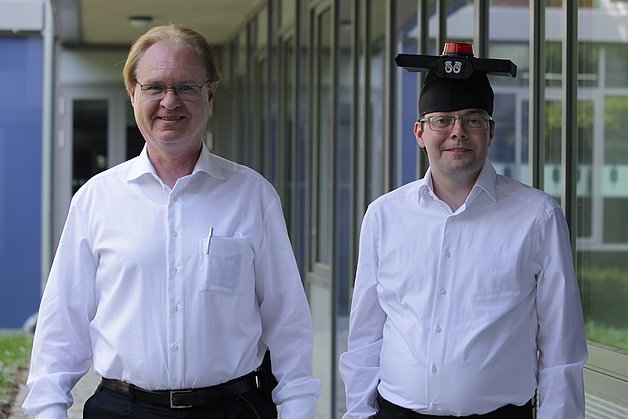
Press release,
Reliable and faster chip designs through invasive and parametric simulation methods
Dissertation on new methods for automation in integrated circuit design
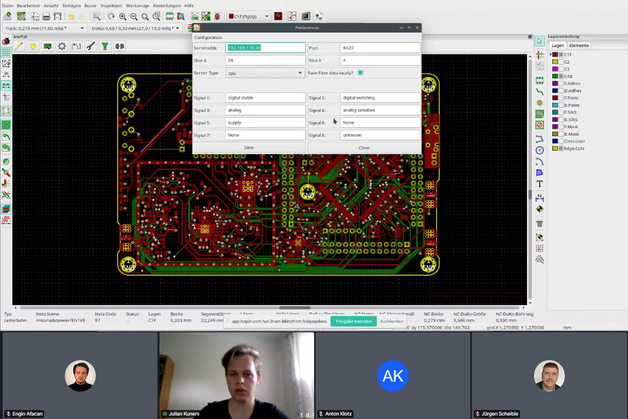
Press release,
EDA Competition Award for "Trash or Treasure" - Intelligent Layout Processing
1st prize of the IEEE CEDA goes to young scientists from Ilmenau TU and IMMS
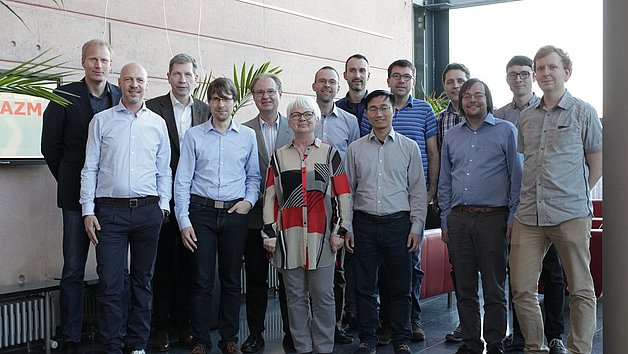
Press release,
Forschergruppe „IntelligEnt“ gestartet: Künstliche Intelligenz und Machine Learning für den Entwurf von Mikroelektronik
IMMS und TU Ilmenau forschen an neuartigen Assistenzsystemen für Chip-Designer
Contact
Contact
Eric Schäfer, M. Sc.
Head of Microelectronics / Branch Office Erfurt
eric.schaefer(at)imms.de+49 (0) 361 663 25 35
Eric Schäfer and his team research Integrated sensor systems, especially CMOS-based biosensors, ULP sensor systems and AI-based design and test automation. The results are being incorporated into research on the lead applications Sensor systems for in-vitro diagnostics and RFID sensor technology. It will assist you with services for the development of Integrated circuits and with IC design methods.
Funding
The project on which these results are based was supported by the German ”Land“ of Thüringen and co-financed by European Union funds within the framework of the European Regional Development Fund (ERDF) under the reference 2017 FE 9044.