Project ProQuaOpt
IMMS is developing an AI-based control system for resource-efficient online optimisation of injection moulding
There is hardly any time for process optimisation during traditional manual machines set-up
Injection molding is the most widespread process to produce plastic parts for practically all application areas. In this situation, high throughput and good quality parts are important for a manufacturer to survive on the market. For this purpose, machines are usually set up by skilled personnel to produce good parts at the beginning of a batch.
To date, this happens once at the start of a batch and the machine then runs with constant parameters. However, if the ambient conditions change (e.g. the air temperature due to open gates), currently no reaction is possible and the risk of faulty parts increases. In addition, the settings are chosen based on experience. Whether these correspond to the optimum operating point in terms of resource consumption with good product quality is unclear.
The goal is to optimise the injection molding process through AI
In the ProQuaOpt project, we aim to apply AI methods for automated productivity and quality optimisation of the injection molding process. To achieve this, we plan to develop a product-process quality control loop that will consist of a subsystem to interact with the injection molding machine, a measuring element for quality inspection and an AI-based controller.
In addition to responding to changing environmental conditions, the control loop also enables continuous optimisation of the process in terms of resource efficiency. When necessary, the controller provides small adjustments to keep the process at the optimum operating point. Internal feedback is given to the controller via the quality inspection module. The adjustments will mimic steps that an expert would also perform.
IMMS develops AI-based control system
In the ProQuaOpt project, IMMS will combine its expertise in signal acquisition and processing for AI applications as well as the development of AI-based predictive models. We will develop a process-optimising control system for both inline and autonomous operation.
Depending on the input parameters, the control system will optimally adjust the settings of the injection molding machine without affecting quality of the produced parts. The system can also respond to external disturbances. This makes the injection molding process more efficient and results in less defective parts.
Acronym / Name:
ProQuaOpt / Productivity and quality optimisation for plastic injection molding processes with AI methodsDuration:2022 – 2025
Application:
|Injection moldingResearch field:Smart distributed measurement and test systems
Related content
Neue Ansätze in der Kunststoffproduktion – Ein KI-basiertes Assistenzsystem für den Spritzgießprozess
Dominik Schraml1. Silvia Krug2.Thüringer Maschinenbautag, Erfurt, 22. Oktober 2024
1Steinbeis Qualitätssicherung und Bilderverarbeitung GmbH, Ilmenau. 2IMMS Institut für Mikroelektronik- und Mechatronik-Systeme gemeinnützige GmbH, Ehrenbergstraße 27, 98693 Ilmenau, Germany.
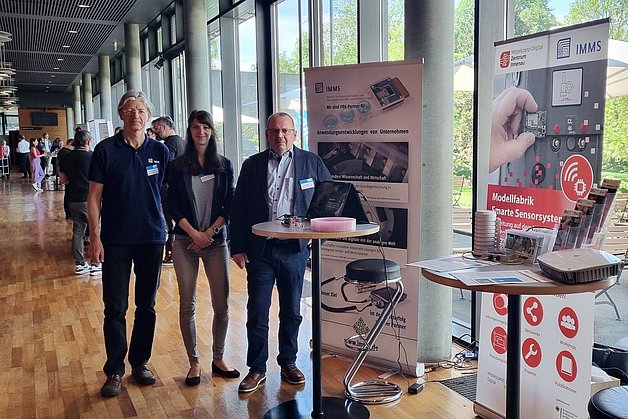
Event,
InnoCON 2025
Innovation policy flagship event of the German Land of Thüringen. Topic “Key technologies: Paving the way for the world of tomorrow“
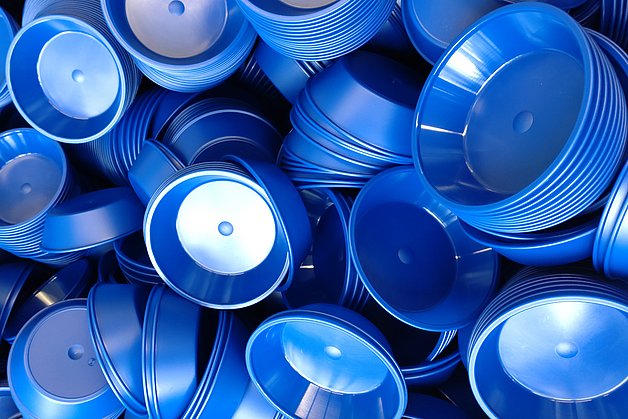
Event,
Jahresauftakt MD & DGQ
Jahresanfangs-Veranstaltung des „Mittelstand-Digital-Zentrums Ilmenau“ mit der Deutschen Gesellschaft für Qualität (DGQ) zur Digitalisierung in der Qualitätssicherung
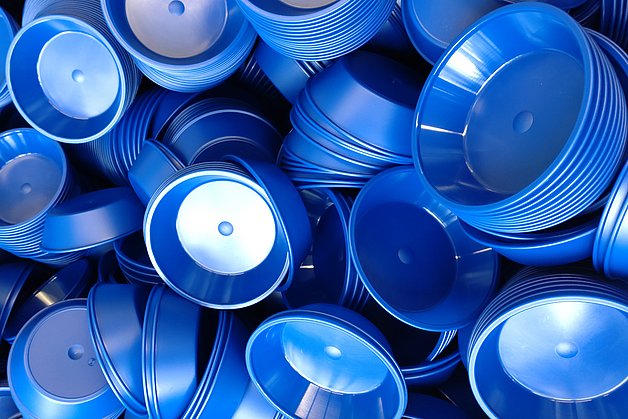
Event,
8. Thüringer Maschinenbautag
Herausforderung Arbeitskräftemangel: Was können Unternehmen tun? Best Practice und neue Wege.
Contact
Contact
Dr.-Ing. Tino Hutschenreuther
Head of System Design
tino.hutschenreuther(at)imms.de+49 (0) 3677 874 93 40
Dr. Tino Hutschenreuther will answer your questions on our research in Smart distributed measurement and test systems and the related core topics Analysis of distributed IoT systems, Embedded AI and Real-time data processing and communications, on the lead applications Adaptive edge AI systems for industrial application and IoT systems for cooperative environmental monitoring as well as on the range of services for the development of embedded systems.
Funding
The ProQuaOpt project on which this report is based was funded by the German Federal Ministry of Education and Research under the reference 01|S22019E. The author is responsible for the content of this publication.