Project AgAVE
Industry 4.0 communications for an assistance system, ML-based, that autonomously analyses connected production chains
The problem: errors are detected in one place but the root cause is often elsewhere
Industrial production chains are organised to operate continuously and with high throughput. Breakdowns and downtime for machinery can lead to high financial losses. It is estimated that the costs of repair and maintenance amount to 15% to 40% of industrial companies’ total indirect costs.
One reason for this is that modern production chains are increasingly complex and composed of many modules or individual machines. With this structure, troubleshooting is very difficult as the location where the error is detected does not necessarily reflect the source location. Consequently, many manual, time-consuming checks are needed to find where the fault originated before resolving it. Intelligent assistance systems (assistants) can help shorten the investigation time and reduce costs.
The solution: local and global ML-based assistants to diagnose root cause
Jointly, Fraunhofer IOSB-INA and IMMS have developed an assistance system for industrial plants. The system automatically detects risks, anomalies, and possible root causes like signs of wear and tear or sensor failures. It gives early warnings to the operator, who receives not only the details of the anomaly noted but also the suggested root cause. This requires a system working on more than one level, because each machine has access to its own data only.
However, to identify the root cause of an error, data from other machines in the production chain is needed as well. Fraunhofer IOSB-INA has developed machine learning (ML) approaches by which each machine is monitored locally and global results, correlations and instructions are derived which the operator can interpret in relation to the complete chain.
The IMMS contribution: Industry 4.0 communications for the different diagnostic assistants
Data has to be exchanged between both monitoring levels. To enable this, we at IMMS developed appropriate communications systems conforming to Industry 4.0 protocols. The systems ensure reliable communication between the local assistants (at machine level) and the global assistants (at the higher analysis level). To achieve this, we defined mechanisms not only to convert data into relevant information but also to interact automatically with further modules in the processing chain. Without such communications, no analysis of the full process is possible. Using Industry 4.0 compliant protocols, ensures that the concept is applicable to any processing chain which applies these standards.
Together the partners implemented the system principles in an application-oriented demonstrator system, comprising communications and analysis components as well as sensor integration. With it, we have shown how the new ML-based assistance system is able to learn decision rules, recognize causal correlation, and identify potential error root causes within a distributed processing chain. It is installed in a production chain for packaging material which is part of the SmartFactoryOWL lab.
We have thus been able to demonstrate that novel applications for automation purposes are possible with using Industry 4.0 communications. The real-life example proves how this communications type opens up the full potential of the novel ML-based methods and the corresponding algorithms for operators of processing chains.
Acronym / Name:
AgAVE / Assistance system for monitoring of networked equipment – The challenge of networking and recognising causal relationshipsDuration:2017 – 2019
Application:
Automation technology and Industry 4.0|Industrial manufacturing processesResearch field:Smart distributed measurement and test systems
Partner
Related content
Fehlerursachenanalyse entlang verteilter Produktionsanlagen mit Hilfe lernender Assistenten
Kaja Balzereit1. Tino Hutschenreuther2.Informationstag “Intelligente Produktionsprozesse: Forschung zu Machine Learning und Künstlicher Intelligenz„, 12. September 2019, Frankfurt am Main
1Fraunhofer-Institut für Optronik, Systemtechnik und Bildauswertung IOSB, Institutsteil Industrielle Automation, Germany. 2IMMS Institut für Mikroelektronik- und Mechatronik-Systeme gemeinnützige GmbH, 98693 Ilmenau, Germany.Gesteigerte Wertschöpfung in modularen Systemen – Fehlerursachenanalyse für Produktionsanlagen
Kaja Balzereit1. Björn Barig2. Alexander Maier1.atp magazin, 8/2019, Vulkan-Verlag
1Fraunhofer IOSB-INA Institutsteil für industrielle Automation, Germany. 2IMMS Institut für Mikroelektronik- und Mechatronik-Systeme gemeinnützige GmbH, 98693 Ilmenau, Germany.Applying OPC-UA for Factory-Wide Industrial Assistance Systems
Björn Barig1. Kaja Balzereit2. Tino Hutschenreuther1.2019 15th IEEE International Workshop on Factory Communication Systems (WFCS), 27 - 29 May 2019, Sundsvall, Sweden, 2019, pp. 1-4. DOI: doi.org/10.1109/WFCS.2019.8757868
1IMMS Institut für Mikroelektronik- und Mechatronik-Systeme gemeinnützige GmbH, 98693 Ilmenau, Germany. 2Fraunhofer IOSB INA, Lemgo, Germany.Assistance Systems for Industrial 4.0 Environments
Björn Barig1.embedded world Conference - Internet of Things - Platforms & Applications, Session 1.3: Internet of Things IV OPC UA I4.0, 26 - 28 February 2019, Nürnberg, Germany
1IMMS Institut für Mikroelektronik- und Mechatronik-Systeme gemeinnützige GmbH, 98693 Ilmenau, Germany.
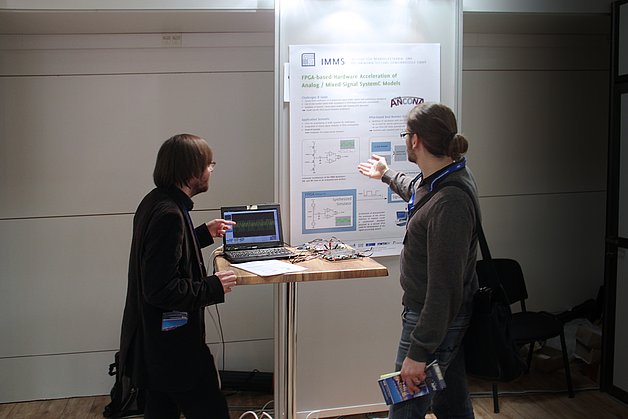
Event,
embedded world 2019
embedded world Conference – Internet of Things - Platforms & Applications track
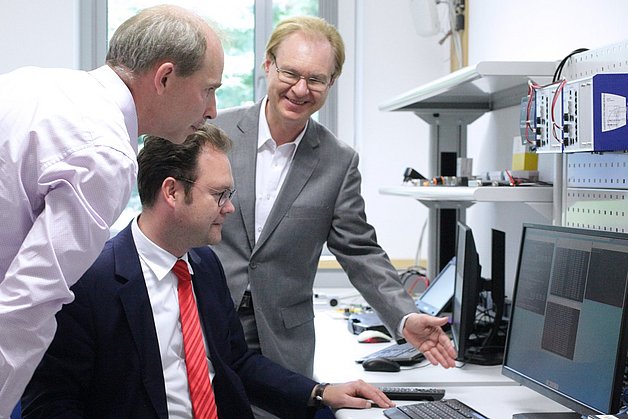
Press release,
Bundestagsabgeordneter Tankred Schipanski zu Besuch am IMMS
Am 12. Juli 2018 besuchte der für den Landkreis Gotha und den Ilm-Kreis gewählte Bundestagsabgeordnete Tankred Schipanski das IMMS. Zur Pressemitteilung auf www.tankred-schipanski.de.
Contact
Contact
Dr.-Ing. Tino Hutschenreuther
Head of System Design
tino.hutschenreuther(at)imms.de+49 (0) 3677 874 93 40
Dr. Tino Hutschenreuther will answer your questions on our research in Smart distributed measurement and test systems and the related core topics Analysis of distributed IoT systems, Embedded AI and Real-time data processing and communications, on the lead applications Adaptive edge AI systems for industrial application and IoT systems for cooperative environmental monitoring as well as on the range of services for the development of embedded systems.
Funding
The AgAVE project was funded by the German Federal Ministry of Economic Affairs and Energy as a project of the Industrial Community Research (IGF) under the reference 19341BG.