IEEE MetroInd4.0&IoT 2024
TEEMSC – Trainable Energy Efficient Machine Diagnosis using Singular Values and Canonical Crosscorrelation
Rick Pandey, Sebastian Uziel, Tino Hutschenreuther, Silvia Krug
Predictive Maintenance (PdM) has been a challenge due to insufficient labelled data and dynamic industrial environments. These problems require a data driven solution for machine degradation analysis which is capable of on-device learning and adapting to the changing industrial environments. Therefore, we propose a new algorithm TEEMSC (Trainable Energy Efficient Machine Diagnosis using Singular Values and Canonical Crosscorrelation) for machine degradation analysis which can be trained completely on a sensor node and the learned parameters are adaptive to dynamic industrial scenarios. TEEMSC learns from unlabelled data in mainly unsupervised manner. In comparison to TEEMSC, the existing data driven unsupervised methods mostly do anomaly detection and the neural network-based solutions either rely upon a cumbersome data acquisition and labelling process or suffer from concept drift caused due to changing environmental scenarios. Considering bearing degradation as a PdM scenario we have tested the algorithm on PRONOSTIA and XJTU bearing dataset and compared performance of TEEMSC with three existing degradation trend analysis methods namely Principal Component Analysis, Power Spectral Density and Kurtosis on the wavelet decomposition of the original vibration signal. Our results can clearly outperform existing unsupervised degradation trend analysis and anomaly detection methods for on-device learning.
Related content
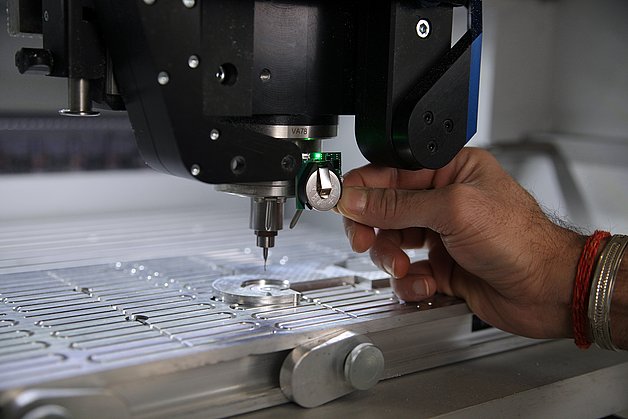
Project
HoLoDEC
IMMS researches ultra-low-power architectures (ULP) and circuit concepts as well as energy-efficient edge-AI systems with overall system energy…
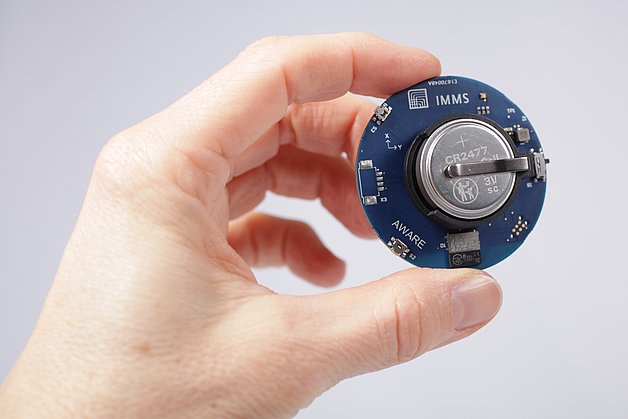
Press release,
AI without the net: Energy-efficient edge AI sensor system for industrial monitoring applications
IMMS exhibits at embedded world
Contact
Contact
Dipl.-Hdl. Dipl.-Des. Beate Hövelmans
Head of Corporate Communications
beate.hoevelmans(at)imms.de+49 (0) 3677 874 93 13
Beate Hövelmans is responsible for the text and image editorial work on this website, for the social media presence of IMMS on LinkedIn and YouTube, the annual reports, for press and media relations with regional and specialist media and other communication formats. She provides texts, photographs and video material for your reporting on IMMS, arranges contacts for interviews and is the contact person for events.